What is MDM Workflow Management?
Tuesday, April 13, 2021 / Updated: August 19, 2022
The process of workflow management was once a little-understood process that has now become an imperative focus for business management across organizations large and small. Business managers look for Master Data Management (MDM) as a means to streamline and standardize the process of workflow management.
In the old days, one might need to get a separate software tool in order to use MDM-based solutions, but today, many workflow management software come with MDM features built-in.v
What Exactly Is Master Data Management?
This is generally a combination of technologies that are used to increase the uniformity of processes, improve the accuracy of data, and contribute to consistency in business processes within an organization.
This is a way to manage master data in a centralized way that follows the strict rules of your strategic goals or operational strategies.
In essence, MDM is a process whereby technology is used to create a master data record that is used across all parts of a company. This ensures that the processes are all using the same, updated, and accurate information. Consistency of data is incredibly important to a wide range of business processes.
Today many people use workflow software that has these tools built-in, but this may not be an option in the system of tools you currently use. Whether you use one of powerful options of CMW Tracker, or another third-party software source, you can easily integrate MDM technologies into the existing system, allowing you further functionality without having to purchase and implement a completely new system or software.
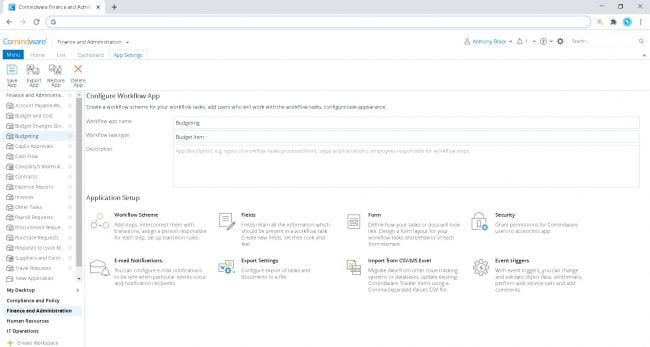
Benefits of MDM Workflow Management
There is a range of benefits associated with MDM. Having a master database of regularly-updated information that all parts of the business users perform their business functions helps to ensure that all parties are using the most current information and that everyone is working from the same set of data.
Having everyone work from a master source of current data helps to eliminate bad data or information that is out of date. It also works to improve the efficiency of processes by ensuring that all parties necessarily have access to the same set of information with ease.
The ability to trust that a master data set is up-to-date and accurate helps the decision-making process as well. You will be using current, accurate data that will allow you to make better and more informed decisions.
Master Data Management also makes it easier to fully comply with the range of compliance and regulatory reporting most businesses are required to do.
In Closing
Better managing workflow across different aspects of a business is an important part of creating a streamlined and successful business. Master Data Management is an excellent way to help improve efficiency, streamline operations, and reduce errors in various aspects of a business’s operations. Some workflow management software already has these tools built-in, but older tools may not but will allow for the integration of third-party software that will provide this functionality.
In essence, MDM allows for the creation of a master set of data that all parties within the organization use for the various needs of the company. This will be a current, accurate, and up-to-date database of information that all parties can use to run processes, inform decision-making, and respond to basic compliance reporting. Everyone working from the same set of consistent data is incredibly useful and will help reduce the likelihood of errors or issues within a given process.
Having a master database of information allows all parties within an organization easy access to the same information and data that can be used for a wide range of business functions. There are a wide range of benefits to the implementation of Master Data Management including increased efficiency and transparency, the ability to provide accurate and up-to-date information across the business as a whole, the ability to access said data remotely, and more.
Whether you are using workflow management tools that have MDM functions built-in, or you are looking at getting a third-party option to integrate within your existing system, this is a really important and useful way to help improve the accuracy and efficiency of processes within pretty much all elements of a business. Using these tools will help aid in a range of important business functions and give you better data that will help you make smarter decisions.
Posted on: in Workflow